Ise AI Insights #09Open source versus proprietary models in Retail visual gen
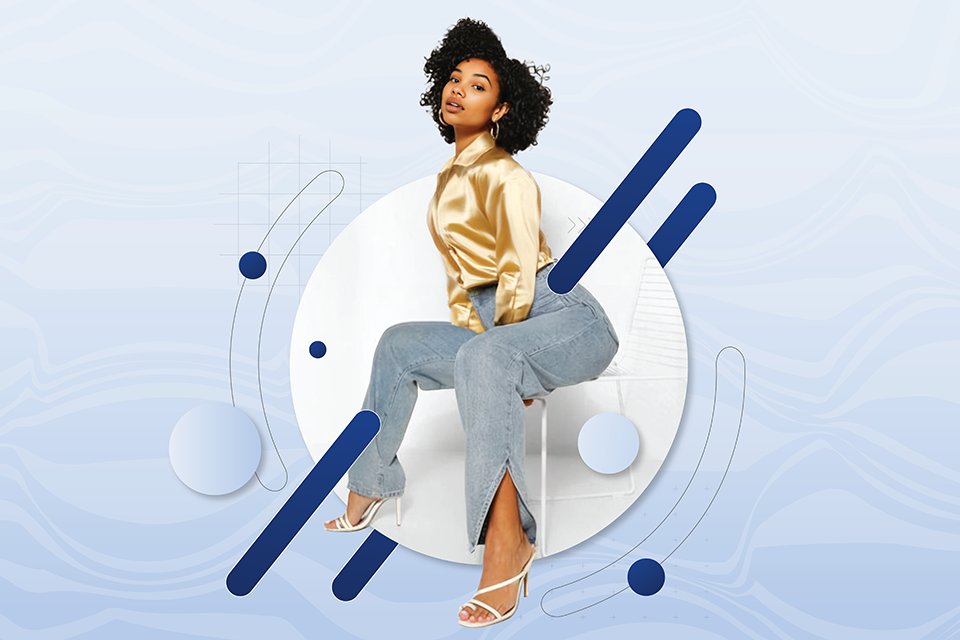
Last week at a conference, Ise AI CEO Vanessa Yan met the Head of Digital of a large omnichannel retailer. The executive mentioned that the retailer has been trying to fine tune open source models (like ones available on Stability AI) but have not been able to achieve desired results. In this article, we will answer two main reasons why finetuning a general purpose model is less ROI-effective than working with a vertical AI company like Ise AI.
👕 Insight 1: Fundamentally different model architecture
As we explained in a previous article linked below, Ise AI’s models have a fundamentally different architecture compared to open source image generation models. General purpose image generation models optimize for creativity and adhering to text prompts across a large variety of use cases, e.g. anime, cartoon. No matter how much you fine tune the open source model, the very first step in the model architecture is already hallucination-prone, so you can’t fine tune it to accuracy.Â
In contrast, Ise AI’s models are purpose-trained with a different set of priorities — namely, fidelity to product specifications and detail preservation. By training our own proprietary foundation model architecture, Ise AI ensures that the generated images not only align with the given prompts but more importantly retain the intricate features of retail products, such as textures, patterns, and packaging details. This level of precision allows retailers to generate high-quality, consistent product images that can be used confidently for e-commerce listings, marketing materials, or inventory management.
In short, Ise AI solves the hallucination issue of generative AI imagery, making it the ideal solution for professionals in the retail industry who rely on visual precision.
💡 Insight 2: No single model gets the job done
In the realm of image generation, particularly with diffusion-based models, it’s important to understand that no single model is perfect for every task. This is fundamentally different from many text-based models, which often can achieve high accuracy across a wide range of language tasks through fine-tuning or prompt engineering.
No single model fits all use cases. No single image generation model can excel across all use cases. A model trained to generate hyper-realistic images of furniture for e-commerce purposes may not perform well when asked to create abstract art or cartoon-ish characters. Similarly, an image model optimizing for flexibility across consumer use cases lacks the precision needed to generate accurate product images for retail.
This leads to the necessity for specialized models in domains like retail, fashion, automotive, etc., where attention to detail and physical constraints is paramount.
💠Insight 3: There is significant know-how on combining the models synergistically
Through our research and development efforts, Ise AI learned that in order to unlock enterprise-quality outputs we need to not only train proprietary domain-specific foundation models for retail, but also weave together multiple models synergistically. Our tech stack actually comprises four different types of models that each specialize in a certain subtask.
Training your own domain-specific models, building the infrastructure to keep multiple models running 24/7 in the background, and having full-time AI research scientists on staff to continuously optimize results is too costly for retail enterprises. Especially with Ise AI’s outcome-based pricing model, brands and retailers often find it more attractive to partner with us and spend their R&D efforts on more straightforward LLM use cases.
Follow us on LinkedIn and Instagram for more Ise AI Insights. Try Ise AI for free today at https://www.ise-ai.com/ or reach out to michelle[at]ise-ai.com for a personalized demo.